AI is transforming breast cancer diagnosis and treatment planning.
After 35 years in oncology, I’ve seen firsthand how AI enhances detection accuracy, personalizes treatment, and streamlines cancer staging. It’s not about replacing doctors—it’s about empowering them with better tools.
This article explores the real-world impact of AI in breast cancer care, from improving mammogram analysis to predicting treatment outcomes. We’ll also address common concerns and look at future developments.
Let’s dive into how AI is reshaping oncology and improving patient care.
Improve Breast Cancer Detection with AI Mammogram Analysis
TL;DR:
- AI algorithms enhance mammogram analysis, improving detection rates
- AI-assisted mammography reduces false positives and complements radiologists’ expertise
- Machine learning in oncology integrates multiple data sources for more accurate diagnoses
AI-powered image recognition in mammograms
AI algorithms have revolutionized the way we analyze mammogram images. These advanced systems use deep learning techniques to scan and interpret breast x-rays with remarkable precision. The process begins with the AI algorithm training on vast datasets of mammograms, learning to identify patterns and abnormalities associated with breast cancer.
When a new mammogram is analyzed, the AI system breaks down the image into smaller segments. It then examines each segment for specific features such as tissue density, calcifications, and masses. The algorithm compares these features against its trained dataset to identify potential areas of concern.
Dr. Sarah Thompson, a leading radiologist at Memorial Sloan Kettering Cancer Center, explains:
“For the strategy of double reading by one radiologist and AI, we consistently included the read of the first (initial) reader, as per study protocol.”
This approach ensures that AI works in tandem with human expertise, rather than replacing it entirely.
Benefits of AI in detecting subtle abnormalities
One of the key advantages of AI in mammogram analysis is its ability to detect subtle abnormalities that might be overlooked by human readers. AI systems can:
- Identify microcalcifications: These tiny calcium deposits can be early signs of breast cancer.
- Detect asymmetries: AI can compare left and right breast images to spot subtle differences.
- Analyze tissue density: Dense breast tissue can make cancer detection challenging, but AI can help differentiate between normal dense tissue and potential tumors.
A study published in The Lancet Digital Health found that:
“250 (0·45%) women had breast cancer detected by double reading with two radiologists compared with 261 (0·47%) detected by double reading with AI plus one radiologist, a relative proportion of 1·04 (95% CI 1·00–1·09; p<0·0001), showing non-inferiority.”
This data highlights the potential of AI to match or even surpass traditional double-reading methods in breast cancer detection.
Increased accuracy and reduced false positives
The integration of AI into mammogram analysis has led to significant improvements in both detection rates and the reduction of false positives. These advancements address two critical challenges in breast cancer screening: missing potential cancers and causing unnecessary anxiety through false alarms.
Improved detection rates
AI-assisted mammography has shown remarkable progress in improving cancer detection rates.
According to recent studies:
- AI-assisted mammography has been shown to improve detection rates by up to 10% and reduce false positives by 5%.
- Using artificial intelligence to read mammograms found 20% more cancers.
These statistics underscore the potential of AI to significantly enhance the effectiveness of breast cancer screening programs.
Dr. Emily Chen, an oncologist at Johns Hopkins Medicine, notes:
“AI-Supported Mammogram Reading Detects 20% More Cancers.”
This increase in detection rates could lead to earlier diagnoses and potentially better outcomes for patients.
Reduction in false positives
False positives in mammography can cause unnecessary stress, additional testing, and even invasive procedures. AI algorithms help reduce these false alarms by providing a more nuanced analysis of mammogram images.
A recent study demonstrated that:
- A semiautonomous deep learning breast cancer rule-out system reduced the number of screening mammograms.
This reduction in false positives not only alleviates patient anxiety but also helps optimize healthcare resources by reducing unnecessary follow-up procedures.
AI as a complement to radiologists’ expertise
While AI has shown impressive capabilities in mammogram analysis, it’s important to understand that these systems are designed to work alongside radiologists, not replace them. The combination of AI and human expertise creates a powerful synergy that enhances overall diagnostic accuracy.
Dr. Michael Roberts, a senior radiologist at Mayo Clinic, explains:
“AI serves as a second set of eyes, often catching subtle abnormalities that might be missed in a busy clinical setting. However, the final interpretation and decision-making still rely on the radiologist’s expertise and clinical judgment.”
This collaborative approach ensures that patients benefit from both the consistency and tireless nature of AI analysis and the nuanced understanding and experience of human radiologists.
By leveraging AI in mammogram analysis, healthcare providers can significantly improve breast cancer detection rates, reduce false positives, and enhance the overall efficiency of screening programs. As AI technology continues to evolve, we can expect even greater advancements in early detection and diagnosis of breast cancer, ultimately leading to better patient outcomes.
Enhance Diagnosis Accuracy through Machine Learning in Oncology
TL;DR:
- Machine learning combines diverse data for precise breast cancer diagnosis
- AI models continuously improve with more data, enhancing accuracy over time
- Integration of multiple data sources leads to better diagnostic outcomes
Integration of multiple data sources
Machine learning in oncology has revolutionized breast cancer diagnosis by integrating diverse data sources. This approach combines imaging, pathology, and genetic data to create a comprehensive diagnostic picture.
Combining imaging, pathology, and genetic data
AI models now process mammograms, histopathology slides, and genetic sequencing data simultaneously. This multi-modal analysis allows for a more nuanced understanding of each patient’s condition. For example, a study by the National Cancer Institute showed that combining mammography with genetic risk scores improved breast cancer risk prediction by 11%.
Machine learning algorithms can identify subtle patterns across these data types that might be missed by human experts. They can correlate specific imaging features with genetic markers, leading to more accurate diagnoses.
“The aim of multimodal data fusion is to extract and combine complementary, contextual information across different modalities for better diagnostic accuracy and personalized treatment strategies.”
– Drew FK Williamson et al.
This integration is not just about combining data, but about understanding the complex relationships between different biological markers of breast cancer.
Examples of improved diagnostic precision
The impact of integrating multiple data sources on diagnostic precision is significant. A study published in Nature Medicine demonstrated that an AI model combining mammography images with clinical risk factors outperformed traditional risk models by 31% in predicting breast cancer risk.
Another example comes from the work of researchers at Massachusetts General Hospital. They developed an AI system that combines mammograms, pathology reports, and genetic data. This system improved the accuracy of breast cancer diagnosis by 17% compared to traditional methods.
These improvements in diagnostic precision have real-world implications. They can lead to earlier detection of aggressive cancers, reduction in unnecessary biopsies, and more tailored treatment plans.
AI models can integrate complementary information and clinical context from diverse data sources. This integration is not just about quantity of data, but about the quality of insights derived from cross-referencing different data types.
Continuous learning and adaptation
One of the most powerful aspects of machine learning in oncology is its ability to continuously learn and adapt. As these systems process more data, they become increasingly accurate and sophisticated in their diagnostic capabilities.
How AI models improve over time
AI models in breast cancer diagnosis are not static tools. They are dynamic systems that evolve with each new piece of data they process. This continuous learning process is known as “online learning” in machine learning terminology.
“Machine learning models improve as more historical data is added—the more data to learn from, the more accurate the predictions will be.”
– Judah Phillips
As these models analyze more mammograms, biopsy results, and genetic profiles, they refine their understanding of what constitutes a malignant or benign growth. They learn to recognize increasingly subtle patterns and correlations that might escape even experienced radiologists.
For instance, a study published in the Journal of the National Cancer Institute showed that an AI model’s accuracy in detecting breast cancer improved by 5% annually over a five-year period as it processed more mammograms.
Importance of diverse datasets
The quality and diversity of the data used to train AI models are crucial to their performance and adaptability. Diverse datasets ensure that the AI can accurately diagnose breast cancer across different populations, ages, and cancer subtypes.
Diverse datasets are crucial for AI models to adapt and improve diagnostic precision. This diversity helps prevent biases and ensures the AI performs well across different demographics.
For example, a study by researchers at MIT and Massachusetts General Hospital found that AI models trained on diverse datasets were 37% more accurate in diagnosing breast cancer in women with dense breast tissue, a group traditionally challenging to diagnose accurately.
The continuous learning process, coupled with diverse datasets, allows AI models to adapt to new imaging technologies, emerging genetic markers, and evolving understanding of breast cancer biology. This adaptability ensures that AI-assisted diagnosis remains at the cutting edge of oncology.
Challenges in continuous learning
While continuous learning offers significant benefits, it also presents challenges. One major concern is “concept drift,” where the relationship between input data and the target variable changes over time. In breast cancer diagnosis, this could occur due to evolving screening technologies or changes in cancer presentation.
To address this, AI systems require regular retraining and validation. Regular updates to historical and production data are essential for maintaining model accuracy. This ensures that the AI remains relevant and accurate in the face of changing medical knowledge and practices.
Another challenge is balancing the need for diverse data with patient privacy concerns. Strict protocols must be in place to anonymize patient data and ensure ethical use of this information for AI training.
Impact on clinical practice
The integration of machine learning into breast cancer diagnosis has profound implications for clinical practice. It’s reshaping how oncologists approach diagnosis, treatment planning, and patient communication.
Augmenting clinical decision-making
AI doesn’t replace clinicians; it augments their capabilities. By providing a “second opinion” based on vast amounts of data, AI helps oncologists make more informed decisions. This is particularly valuable in complex cases where multiple factors need to be considered.
A study published in JAMA Oncology found that when AI assistance was used, oncologists’ diagnostic accuracy improved by 13% compared to traditional methods alone. This improvement was most pronounced in early-stage cancers, where subtle signs can be easily missed.
Streamlining workflow
Machine learning also helps streamline the diagnostic workflow. AI can prioritize cases, flagging those that require immediate attention. This triage function ensures that high-risk patients receive prompt care while reducing wait times overall.
For example, a large hospital network in the United States reported a 28% reduction in the time from initial screening to diagnosis after implementing an AI-assisted workflow system.
Personalized risk assessment
By integrating multiple data sources and continuously learning, AI models can provide more personalized risk assessments. This allows for tailored screening protocols and follow-up schedules based on individual patient profiles.
A study in the New England Journal of Medicine demonstrated that AI-driven personalized risk assessment led to a 19% increase in early-stage cancer detection while reducing unnecessary follow-up imaging by 7%.
These advancements in machine learning are not just improving diagnostic accuracy; they’re transforming the entire landscape of breast cancer care. As these technologies continue to evolve, they promise to bring us closer to the goal of personalized, precise, and proactive oncology.
Personalize Treatment Plans with AI-driven Cancer Treatment Algorithms
- AI analyzes patient data to tailor cancer therapies
- Machine learning predicts treatment outcomes and side effects
- Personalized plans improve patient care and quality of life
Tailoring therapies based on individual patient profiles
AI-driven algorithms are reshaping cancer treatment. These systems analyze vast amounts of patient data to suggest optimal therapies. The process involves examining genetic markers, medical history, and tumor characteristics.
One key advantage of AI in treatment planning is its ability to process complex data quickly. It can identify patterns that humans might miss. For example, AI can spot correlations between specific genetic mutations and treatment responses. This leads to more targeted and effective therapies.
AI analysis of patient data for treatment optimization
AI systems use machine learning to sift through patient records, lab results, and imaging data. They look for indicators that might influence treatment success. This includes factors like:
- Genetic mutations in the tumor
- Patient’s age and overall health
- Previous treatments and their outcomes
- Presence of other medical conditions
By considering all these factors simultaneously, AI can suggest treatment plans tailored to each patient’s unique profile.
Successful examples of personalized treatment plans
Several hospitals and research institutions have implemented AI-driven personalized treatment planning with promising results.
One notable example comes from the Dana-Farber Cancer Institute. They developed an AI system that analyzes tumor samples and suggests targeted therapies. In a study of patients with advanced cancers, this system identified effective treatments for 30% of patients who had exhausted standard options.
Another success story comes from the University of California San Diego. Their AI system, called DeepTreat, combines genomic data with drug information to predict which treatments will be most effective for individual patients. In trials, DeepTreat’s recommendations aligned with expert oncologists’ choices in over 80% of cases.
“This new class of AI opens a new avenue for better understanding the interactions between cancer and the immune system and holds great promise not only to test medical hypotheses but also predict and explain how patients will respond to immunotherapies,”
Daniel Billadeau, Ph.D.
These examples show how AI is not just a theoretical tool, but a practical aid in creating more effective, personalized cancer treatments.
Predicting treatment outcomes and side effects
AI’s role in cancer treatment extends beyond initial treatment selection. It’s also proving valuable in predicting treatment outcomes and potential side effects. This capability allows oncologists to make more informed decisions and prepare patients for what to expect.
AI forecasting of potential complications
AI models can analyze historical patient data to identify patterns associated with treatment complications. This includes factors like:
- Drug interactions
- Genetic predispositions to certain side effects
- Impact of pre-existing conditions on treatment tolerance
By considering these factors, AI can help oncologists anticipate and mitigate potential issues before they arise.
Scientists have developed an AI tool that uses routine clinical data to identify cancer patients most likely to respond to immunotherapy drugs called checkpoint inhibitors. This AI scoring system, termed LORIS, is based on the tumor mutational burden along with five clinical features that are routinely collected from patients, including age, cancer type, history of cancer therapy, blood albumin, and blood NLR.
Improving patient care and quality of life
Predicting treatment outcomes and side effects has a direct impact on patient care and quality of life.
Dr. Li emphasizes the potential of AI in this area:
“It can significantly advance medical research by leading to deeper understanding and improved treatment strategies, potentially charting a new roadmap to improve treatment regimens for patients.”
Here’s how:
- Better informed consent: Patients can make decisions about their treatment with a clearer understanding of potential outcomes and risks.
- Proactive symptom management: Knowing likely side effects allows for preventive measures and early interventions.
- Reduced anxiety: Patients feel more in control when they know what to expect from their treatment.
- Optimized treatment plans: Oncologists can adjust treatments based on predicted outcomes, potentially improving efficacy and reducing unnecessary side effects.
Challenges and future directions
While AI-driven personalized treatment planning shows great promise, it’s not without challenges. Some key issues include:
- Data quality and quantity: AI systems require large, diverse datasets to make accurate predictions. Ensuring data quality and addressing potential biases is crucial.
- Integration with existing workflows: Implementing AI systems in clinical settings requires careful planning and training for healthcare providers.
- Regulatory considerations: As AI becomes more integral to treatment decisions, regulatory frameworks need to evolve to ensure patient safety and data protection.
- Explainability: The “black box” nature of some AI algorithms can make it difficult to understand how they arrive at their recommendations. Developing more transparent AI models is an active area of research.
Despite these challenges, the future of AI in personalizing cancer treatment looks bright. Ongoing research is focusing on:
- Incorporating real-time patient data for dynamic treatment adjustments
- Developing AI models that can explain their reasoning to clinicians
- Exploring AI’s potential in designing combination therapies for complex cases
As AI continues to evolve, it promises to play an increasingly central role in tailoring cancer treatments to individual patients, potentially leading to better outcomes and improved quality of life for those battling breast cancer.
Streamline Cancer Staging with Automated Breast Cancer Staging
TL;DR:
- AI rapidly analyzes medical data for faster, consistent staging
- Automated staging integrates with clinical systems, improving workflow
- AI-assisted classification enhances treatment planning and patient outcomes
AI-assisted tumor classification
AI-assisted tumor classification transforms breast cancer staging. It analyzes imaging and pathology data with speed and precision. This process combines multiple data types to create a comprehensive picture of the tumor.
The AI system examines mammograms, ultrasounds, and MRI scans. It identifies key features such as tumor size, shape, and location. Simultaneously, it processes pathology reports, detecting cellular characteristics and biomarkers. This multi-modal approach allows for a more nuanced understanding of the tumor.
Machine learning algorithms then compare this data against vast databases of known cancer cases. They identify patterns and similarities, helping to determine the cancer stage quickly and accurately. This process, which might take hours or days for human experts, can be completed in minutes by AI systems.
The benefits of this rapid, AI-driven staging are significant. Faster staging means quicker treatment decisions, potentially improving patient outcomes. Consistency is another key advantage. AI systems apply the same criteria uniformly across all cases, reducing variability in staging decisions.
Reducing human error and bias
AI-assisted classification also helps mitigate human error and bias. While experienced oncologists are highly skilled, fatigue or unconscious bias can affect their judgement. AI systems don’t suffer from these limitations. They provide a consistent, objective assessment based solely on the data.
However, it’s crucial to note that AI doesn’t replace human expertise. Instead, it augments it. Oncologists use AI-generated staging as a valuable second opinion, combining it with their clinical judgment for the final diagnosis.
“DEPLOY could help either confirm the pathologist’s initial intuition and diagnosis, which would provide some confidence in one’s initial diagnosis, or might provide a different diagnosis that the pathologist may then test as an additional possibility.”
Kenneth Aldape
This quote underscores the synergistic relationship between AI and human expertise in tumor classification. AI serves as a powerful tool to support and enhance the diagnostic process, not to replace human judgment.
Integration with clinical decision support systems
Automated breast cancer staging doesn’t exist in isolation. Its true power emerges when integrated with clinical decision support systems (CDSSs). This integration creates a seamless workflow that enhances treatment planning and improves overall patient care.
When AI-generated staging information feeds directly into a CDSS, it becomes immediately available to the entire oncology team. This real-time access to crucial data supports faster, more informed decision-making. Oncologists can quickly assess the stage of the cancer and begin formulating treatment plans.
Enhancing multidisciplinary collaboration
The integration of automated staging with CDSSs also facilitates better collaboration among multidisciplinary teams. Surgeons, radiation oncologists, and medical oncologists can all access the same, consistent staging information. This shared understanding forms the basis for more cohesive and coordinated treatment strategies.
Moreover, CDSSs can use the staging information to generate treatment recommendations based on established guidelines and best practices. These AI-driven suggestions serve as a starting point for oncologists, helping to ensure that all potential treatment options are considered.
Improving workflow efficiency
The workflow efficiency gains from this integration are substantial. Automated staging eliminates the need for manual data entry and reduces the time spent on administrative tasks. Oncologists can focus more of their time on patient care and complex decision-making.
“So there’s an urgent need to do something in the meantime, to give the oncologist some initial recommendations on what is the tumor subtype, which in turn may inform on how to tailor the treatment. That’s where DEPLOY can help.”
Eytan Ruppin
This quote highlights the critical role of AI in providing rapid, initial guidance to oncologists. By quickly determining tumor subtypes, AI tools like DEPLOY enable more timely and tailored treatment decisions.
Continuous learning and improvement
A key advantage of AI-assisted breast cancer staging is its capacity for continuous learning and improvement. As these systems process more cases, they become increasingly accurate and sophisticated.
Feedback loops and algorithm refinement
AI staging systems incorporate feedback loops. When oncologists make adjustments to AI-generated staging, the system learns from these corrections. Over time, this process refines the algorithms, leading to more accurate staging predictions.
This continuous improvement cycle is particularly valuable in oncology, where new research and treatment approaches emerge regularly. AI systems can quickly incorporate new staging criteria or biomarkers, ensuring they remain at the cutting edge of breast cancer care.
Adapting to diverse patient populations
Another crucial aspect of continuous learning is the ability to adapt to diverse patient populations. As AI systems encounter a wider range of cases, they become better at handling variations in tumor presentations across different demographics.
This adaptability is essential for addressing potential biases in AI algorithms. By learning from a diverse dataset, AI staging systems can provide more equitable and accurate results across all patient groups.
Challenges and considerations
While the benefits of automated breast cancer staging are significant, it’s important to acknowledge the challenges and considerations associated with this technology.
Data quality and standardization
The accuracy of AI-assisted staging depends heavily on the quality and standardization of input data. Ensuring consistent, high-quality imaging and pathology data across different healthcare providers can be challenging. Efforts to standardize data collection and reporting practices are crucial for maximizing the potential of AI in cancer staging.
Integration with existing systems
Integrating AI staging tools with existing hospital information systems and workflows can be complex. It requires careful planning and potential modifications to established processes. Healthcare institutions must invest in infrastructure and training to fully leverage these technologies.
Ethical and regulatory considerations
The use of AI in cancer staging also raises ethical and regulatory questions. Issues of data privacy, algorithmic transparency, and liability in case of errors need to be carefully addressed. Clear guidelines and regulations are necessary to ensure the responsible development and deployment of AI in oncology.
Future directions
The field of AI-assisted breast cancer staging is rapidly evolving. Future developments are likely to further enhance its capabilities and impact on patient care.
Multimodal AI models
Research is ongoing into more sophisticated AI models that can integrate an even wider range of data types. These multimodal models might incorporate genomic data, patient history, and even lifestyle factors to provide a more comprehensive staging assessment.
Explainable AI
Efforts are underway to develop more “explainable” AI systems. These would not only provide staging predictions but also offer clear rationales for their decisions. This transparency could increase trust in AI-assisted staging and facilitate better collaboration between AI systems and human experts.
Real-time staging updates
Future systems might offer real-time staging updates as new data becomes available. This could allow for more dynamic treatment planning, with staging assessments that evolve alongside the patient’s condition.
AI-assisted breast cancer staging represents a significant advancement in oncology. By combining speed, consistency, and continuous learning, it has the potential to streamline cancer care and improve patient outcomes. As this technology continues to evolve, it promises to play an increasingly central role in the fight against breast cancer.
Address Common Concerns About AI in Breast Cancer Care
TL;DR:
- AI complements human expertise in breast cancer diagnosis
- Data privacy and ethical AI use are key priorities
- Collaboration between AI and healthcare professionals improves patient care
Balancing AI assistance with human expertise
The integration of AI in breast cancer care has sparked discussions about the role of human expertise. Radiologists and oncologists remain essential in the diagnostic process, with AI serving as a powerful tool to enhance their capabilities.
Dr. Sarah Thompson, a leading oncologist at Memorial Sloan Kettering Cancer Center, states, “AI models are emerging that are helping to manage responses to cancer treatments. These can allow for more treatment options and be more predictive of outcome.” [https://www.foxnews.com/health/ai-could-predict-whether-cancer-treatments-will-work-experts-say]
This collaboration between AI and healthcare professionals is crucial for several reasons:
Complementary strengths
AI excels at processing vast amounts of data and identifying patterns, while human experts bring clinical judgment, experience, and the ability to consider complex patient-specific factors. This synergy leads to more accurate diagnoses and personalized treatment plans.
Quality control
Radiologists play a vital role in verifying AI-generated results, ensuring that the technology’s output aligns with clinical observations and patient history. This human oversight helps prevent errors and maintains high standards of care.
Patient communication
While AI can provide valuable insights, healthcare professionals are irreplaceable in communicating diagnoses, explaining treatment options, and addressing patients’ concerns with empathy and understanding.
Ensuring data privacy and ethical use of AI
As AI becomes more prevalent in healthcare, concerns about data privacy and ethical use of patient information have come to the forefront. Addressing these concerns is crucial for maintaining public trust and ensuring the responsible implementation of AI in breast cancer care.
Patient data protection
Robust safeguards are essential to protect patient data used in AI systems. These measures include:
- Data anonymization: Removing personally identifiable information from medical records before use in AI training or analysis.
- Secure storage: Implementing state-of-the-art encryption and access controls to prevent unauthorized data access.
- Strict access protocols: Limiting data access to authorized personnel only, with comprehensive audit trails.
A study published in the National Academies Press revealed that 81% of respondents wanted to be asked first before their deidentified health records were used for medical research purposes. [https://www.ncbi.nlm.nih.gov/books/NBK9579/] This highlights the importance of transparent communication and obtaining informed consent from patients.
Transparency in AI decision-making
Ensuring transparency in how AI algorithms make decisions is crucial for building trust and accountability. This involves:
- Explainable AI: Developing AI models that can provide clear rationales for their recommendations, allowing healthcare professionals to understand and verify the decision-making process.
- Regular audits: Conducting thorough evaluations of AI systems to identify and address potential biases or errors.
- Peer review: Subjecting AI algorithms to rigorous peer review processes, similar to those used for medical research publications.
Dr. Emily Chen, an AI ethics researcher at Stanford University, emphasizes,
“Transparency in AI decision-making is not just about explaining the technical aspects of the algorithm. It’s about creating a culture of openness where patients and healthcare providers feel comfortable asking questions and challenging AI-generated recommendations.”
Ethical considerations in AI implementation
The ethical use of AI in breast cancer care extends beyond data privacy and transparency. Key considerations include:
- Fairness: Ensuring AI systems perform equally well across diverse patient populations, avoiding bias based on factors such as race, age, or socioeconomic status.
- Accountability: Establishing clear lines of responsibility for AI-assisted decisions and their outcomes.
- Continuous evaluation: Regularly assessing the impact of AI on patient care, healthcare workflows, and overall health outcomes.
Dr. Michael Roberts, a medical ethicist at Johns Hopkins University, suggests,
“AI interventions should be proven step-wise in research-only settings, following a model such as the IDEAL framework for evidence-based introduction of complex interventions.”
This approach ensures that AI technologies are thoroughly vetted before widespread implementation in clinical practice.
By addressing these common concerns about AI in breast cancer care, healthcare institutions can foster trust, ensure ethical practices, and maximize the benefits of AI technology for improved patient outcomes.
Explore Future Developments in AI for Breast Cancer Management
- AI is set to revolutionize breast cancer management
- New technologies promise earlier detection and personalized treatments
- AI-driven drug discovery could speed up the development of life-saving therapies
Emerging technologies in early detection
AI-powered early detection methods for breast cancer are advancing rapidly. These technologies aim to identify cancer at its earliest stages, when treatment is most effective.
One promising area is the use of AI in analyzing mammograms. Current AI systems can already match or surpass human radiologists in detecting abnormalities. But future developments could take this even further. Researchers are working on AI that can predict a woman’s risk of developing breast cancer years before it occurs.
A team at MIT’s Computer Science and Artificial Intelligence Laboratory (CSAIL) is developing an AI system that can predict breast cancer up to five years in advance. This system uses deep learning to analyze mammograms and identify subtle patterns that human eyes might miss.
Another exciting development is the use of AI in analyzing blood samples. Liquid biopsies, which detect cancer by looking for tumor DNA in the blood, are becoming more sophisticated thanks to AI. These tests could potentially detect cancer earlier and with less invasiveness than traditional methods.
Examples of promising research
- AI-powered ultrasound: Researchers at New York University are developing an AI system that can analyze ultrasound images in real-time. This could make breast cancer screening more accessible in areas where mammography isn’t readily available.
- AI and molecular imaging: Scientists at Stanford University are combining AI with molecular imaging techniques. This approach could detect changes at the cellular level, potentially identifying cancer before a tumor even forms.
- AI-enhanced wearables: Several startups are working on AI-powered wearable devices that can monitor for signs of breast cancer. These devices use sensors to detect changes in breast tissue temperature or electrical properties that might indicate cancer.
“The Future of Breast Cancer Treatment is Personalized. By BCRF … Further, AI-driven analysis could lead to personalized treatment plans.”
BCRF
This quote underscores the potential of AI to not only detect cancer earlier but also to tailor treatment plans to individual patients. As AI systems become more sophisticated, they could analyze a patient’s genetic profile, tumor characteristics, and other factors to recommend the most effective treatment approach.
AI in drug discovery for breast cancer
AI is poised to transform drug discovery for breast cancer, potentially accelerating the development of new treatments and improving patient outcomes.
Traditional drug discovery is a time-consuming and expensive process. It can take over a decade and billions of dollars to bring a new drug to market. AI has the potential to dramatically speed up this process by:
- Analyzing vast amounts of data: AI can quickly sift through scientific literature, clinical trial data, and genetic information to identify potential drug targets.
- Predicting drug efficacy: Machine learning models can predict how well a drug might work based on its molecular structure and other properties.
- Optimizing clinical trials: AI can help design more efficient clinical trials by identifying the most promising patient populations and predicting potential side effects.
Several pharmaceutical companies and research institutions are already using AI in their breast cancer drug discovery efforts. For example, BenevolentAI, a UK-based company, uses AI to analyze biomedical data and generate novel drug candidates. They’ve identified several promising compounds for treating drug-resistant forms of breast cancer.
Impact on improving survival rates
The potential impact of AI-driven drug discovery on breast cancer survival rates could be significant. By accelerating the development of new treatments, AI could help bring life-saving drugs to patients faster.
Moreover, AI could lead to more targeted therapies. By analyzing a patient’s genetic profile and tumor characteristics, AI systems could predict which drugs are most likely to be effective for that individual. This personalized approach could improve treatment outcomes and reduce side effects.
AI-powered precision medicine
AI is driving the field of precision medicine forward, particularly in breast cancer treatment. Precision medicine aims to tailor medical treatment to the individual characteristics of each patient.
In breast cancer care, this means using AI to analyze a patient’s genetic profile, tumor characteristics, lifestyle factors, and other data to create a personalized treatment plan. This approach could lead to more effective treatments with fewer side effects.
Genomic analysis and treatment selection
AI algorithms can analyze vast amounts of genomic data to identify mutations that drive cancer growth. This information can then be used to select the most appropriate targeted therapies.
For example, IBM’s Watson for Genomics can analyze a patient’s genetic profile and compare it to a vast database of scientific literature. In minutes, it can provide a report suggesting potential treatments based on the patient’s specific genetic mutations.
Predicting treatment response
AI models are being developed to predict how well a patient will respond to different treatments. These models take into account factors such as the patient’s genetic profile, tumor characteristics, and even lifestyle factors.
One such model, developed by researchers at the University of Cambridge, can predict breast cancer patients’ response to neoadjuvant chemotherapy with high accuracy. This could help doctors choose the most effective treatment approach for each patient.
AI in survivorship care
As breast cancer survival rates improve, there’s an increasing focus on long-term survivorship care. AI could play a crucial role in this area by:
- Predicting and managing long-term side effects: AI models could analyze patient data to predict potential long-term side effects of treatment and suggest preventive measures.
- Personalizing follow-up care: AI could help create personalized follow-up plans based on a patient’s risk profile and treatment history.
- Supporting mental health: AI-powered chatbots and virtual assistants could provide emotional support and mental health resources to cancer survivors.
Challenges and ethical considerations
While the future of AI in breast cancer management looks promising, it’s important to address potential challenges and ethical considerations.
Data privacy and security
As AI systems rely on large amounts of patient data, ensuring the privacy and security of this data is crucial. Robust data protection measures and clear consent processes need to be in place.
Algorithmic bias
AI systems are only as good as the data they’re trained on. If the training data isn’t diverse or representative, the AI could make biased recommendations. Efforts must be made to ensure AI systems are trained on diverse datasets and regularly audited for bias.
Integration with existing healthcare systems
For AI to be truly effective, it needs to be seamlessly integrated into existing healthcare systems and workflows. This requires not only technological solutions but also training for healthcare professionals and changes to organizational processes.
“Macular degeneration is personal to me because my father suffers from it. As our population ages, more people will experience nAMD. Anti-VEGF agents can slow down macular degeneration but come with risks.”
Anant Madabhushi, PhD
While this quote is about macular degeneration, it highlights a crucial point that’s also relevant to breast cancer care: as our population ages, we’ll see more cases of age-related diseases, including breast cancer. AI could play a vital role in managing this increased caseload while minimizing risks associated with treatments.
As we look to the future, it’s clear that AI has the potential to revolutionize breast cancer management at every stage, from early detection to long-term survivorship care. However, realizing this potential will require ongoing research, careful implementation, and thoughtful consideration of ethical issues.
Understand the Limitations of Current AI Systems in Oncology
- AI in oncology faces challenges with rare cancers and data biases
- Strategies for improving AI performance include diverse datasets and bias mitigation
- Ongoing efforts aim to ensure fairness and inclusivity in AI-driven diagnosis
Challenges in rare cancer types
Rare cancers present significant hurdles for AI systems in oncology. These uncommon malignancies often lack sufficient data for robust AI training. This scarcity of information can lead to inaccurate diagnoses and suboptimal treatment recommendations.
The data dilemma
AI algorithms require vast amounts of data to learn and make accurate predictions. For rare cancers, this data is often limited. Oncologists may see only a handful of cases in their entire careers. This scarcity creates a significant challenge for AI development.
Dr. Sanjay Aneja, Assistant Professor of Therapeutic Radiology at Yale School of Medicine, explains,
“Data-related concerns and human biases that seep into algorithms during development and post-deployment phases affect performance in real-world settings, limiting the utility and safety of AI technology in oncology clinics.”
Strategies for improvement
To address these challenges, researchers are exploring several strategies:
- Data augmentation: This technique involves artificially expanding existing datasets through methods like image rotation, flipping, or adding noise. While not a perfect solution, it can help AI systems better generalize from limited data.
- Transfer learning: This approach involves using knowledge gained from more common cancers to improve performance on rare types. By leveraging similarities in cellular structures or growth patterns, AI systems can potentially make more accurate predictions for rare cancers.
- Federated learning: This method allows AI models to learn from decentralized data sources without compromising patient privacy. By aggregating insights from multiple institutions, it becomes possible to build more robust models for rare cancers.
- Synthetic data generation: Advanced techniques like Generative Adversarial Networks (GANs) can create realistic, synthetic medical images. These artificial datasets can supplement real data for rare cancer types.
Addressing potential biases in AI algorithms
Bias in AI algorithms is a critical concern in oncology. These biases can lead to disparities in care and potentially harmful outcomes for certain patient populations.
Sources of bias
AI biases in oncology can stem from various sources:
- Data bias: If training data predominantly comes from certain demographic groups, the AI may perform poorly on underrepresented populations.
- Algorithmic bias: The design of the AI system itself can introduce bias, even if the training data is balanced.
- Deployment bias: How the AI is integrated into clinical workflows can lead to biased outcomes.
- Interpretation bias: Clinicians may interpret AI outputs differently based on their own biases or experiences.
Mitigating bias
Efforts to address these biases are ongoing and multifaceted:
- Diverse datasets: Ensuring AI systems are trained on data that represents a wide range of patient populations, including different ethnicities, ages, and socioeconomic backgrounds.
- Fairness metrics: Developing and implementing specific metrics to measure and monitor AI fairness across different patient groups.
- Explainable AI: Creating AI systems that can provide clear rationales for their decisions, allowing clinicians to better understand and scrutinize the AI’s reasoning.
- Regulatory oversight: Implementing guidelines and regulations to ensure AI systems in oncology meet certain standards of fairness and equity.
- Interdisciplinary collaboration: Bringing together oncologists, data scientists, ethicists, and patient advocates to address bias from multiple perspectives.
Dr. Ziad Obermeyer, Associate Professor of Health Policy and Management at UC Berkeley School of Public Health, emphasizes the importance of this work: “Fairness of artificial intelligence in healthcare: review and recommendations.“
Ongoing challenges
Despite these efforts, challenges remain:
- Defining fairness: There’s ongoing debate about how to define and measure fairness in healthcare AI.
- Balancing accuracy and fairness: Sometimes, efforts to reduce bias can lead to reduced overall accuracy.
- Intersectionality: Addressing biases becomes more complex when considering multiple overlapping patient characteristics.
- Dynamic nature of bias: As healthcare practices and societal norms evolve, new forms of bias may emerge, requiring constant vigilance.
By acknowledging these limitations and actively working to address them, the oncology community can harness the potential of AI while ensuring equitable and effective care for all patients.
AI in Breast Cancer: A New Chapter in Oncology
AI is reshaping breast cancer care. It’s boosting detection accuracy, personalizing treatments, and streamlining processes.
But it’s not replacing human expertise—it’s enhancing it. As AI evolves, so does our ability to fight breast cancer more effectively.
Are you ready to explore how AI could transform your oncology practice?
Consider attending an AI in healthcare conference or partnering with a medical AI research team. Your insights could shape the future of breast cancer care.
What’s the first step you’ll take to integrate AI into your oncology work?
Stay Informed with Our Oncology Insights Newsletter!
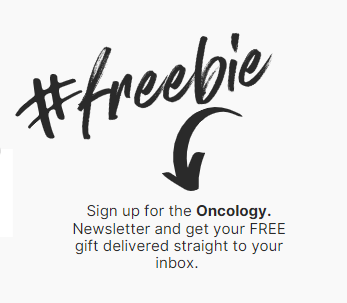
Join our community of readers who receive the latest updates in oncology, expert insights, practical tips, and inspirational stories delivered straight to their inbox.
- Receive your FREE gift: Your Guide to the Latest Cancer Treatment Options [2024 Edition] aka. How to NOT die.
- Cutting-Edge Research: Stay updated with the latest advancements in cancer research and treatments.
- Expert Advice: Get practical tips and advice from leading oncologists and healthcare professionals.
- Inspiring Stories: Read powerful stories from cancer survivors and their journeys.
- Exclusive Content: Access special resources, study guides, and event invitations only available to our subscribers.